As artificial intelligence (AI) continues to reshape industries, OpenAI has established itself as the leading API provider with solutions such as ChatGPT and DALL-E. However, to fully integrate OpenAI's services into business operations, an in-depth understanding of the total cost of ownership (TCO) of these AI initiatives is essential. In order to maximize return on investment (ROI), organizations must consider a wealth of factors including subscription and variable costs.
In this article, we will delve into the various components that contribute to the total cost of ownership (TCO) associated with OpenAI. The article presents both a conceptual framework and a practical case study estimating the TCO of an edtech company chatbot. In particular, we will present a detailed cost structure of an AI assistant designed to embed user queries, retrieve old chats from Pinecone VectorDB, and then utilize the retrieved data, chat history, and user query as input for the generative model to produce a response.
OpenAI and Total Cost of Ownership (TCO)
The rise of OpenAI
According to Sam Altman, OpenAI is on track to hit $1 billion in revenue by 2024. If you’re reading this article, chances are you’re already contributing to that target and wondering if you can optimize your spend.
OpenAI has a comprehensive product suite, one of the standout offerings being ChatGPT. This solution, based on the GPT framework, is designed for engaging and interactive conversations. It has been instrumental in powering chatbots, enabling them to support context-aware dialogues, understand complex prompts, and deliver relevant responses.
The impact of ChatGPT has been profound, as it reached over 100 million active users in January '23, barely two months after launch. This makes it the fastest-adopted consumer application ever, surpassing previous records set by platforms such as Instagram (2.5 years) and Facebook (4.5 years).

Beyond its conversational models, OpenAI has expanded its offerings with tools such as Whisper and DALL-E. Whisper is an automatic speech recognition system that translates spoken language into written text, while DALL-E generates images based on text descriptions.
The notion of TCO in AI
Total Cost of Ownership (TCO) provides a complete view of all costs associated with a product or system, making it a valuable tool for benchmarking projects or services that may not appear comparable at first glance.
For instance, let's say you're considering which of two projects to pursue. The first project costs a lot upfront but is cheaper to maintain, while the other one is inexpensive at the start but then requires expensive regular updates. TCO helps you compare these projects by looking beyond upfront costs, which can be deceiving.
In the AI space, TCO is more than just the visible costs of subscription and usage fees. In fact, it includes other expenses such as AI system integration, staff training, software maintenance, and future training and upgrades. By taking into account all these factors, organizations can make well-informed decisions about their AI investments and effectively track costs over time.
Delving into OpenAI's pricing models
OpenAI's pricing is easy to understand and flexible to meet different needs. OpenAI uses a pay-as-you-go system with costs varying from service to service, so that users pay only for actual consumption. Upon signing up, users get a $5 credit valid over the first three months.
Prices vary by service. Language APIs are priced based on the model selected (larger models = higher price) and on the number of input and output tokens. For reference, one token is roughly equivalent to four characters or 0.75 words in English.
Image generation models such as DALL-E are instead priced by image resolution (higher resolution = higher price). Finally, audio models such as Whisper are priced based on the length of the audio being processed (longer audios = higher price).
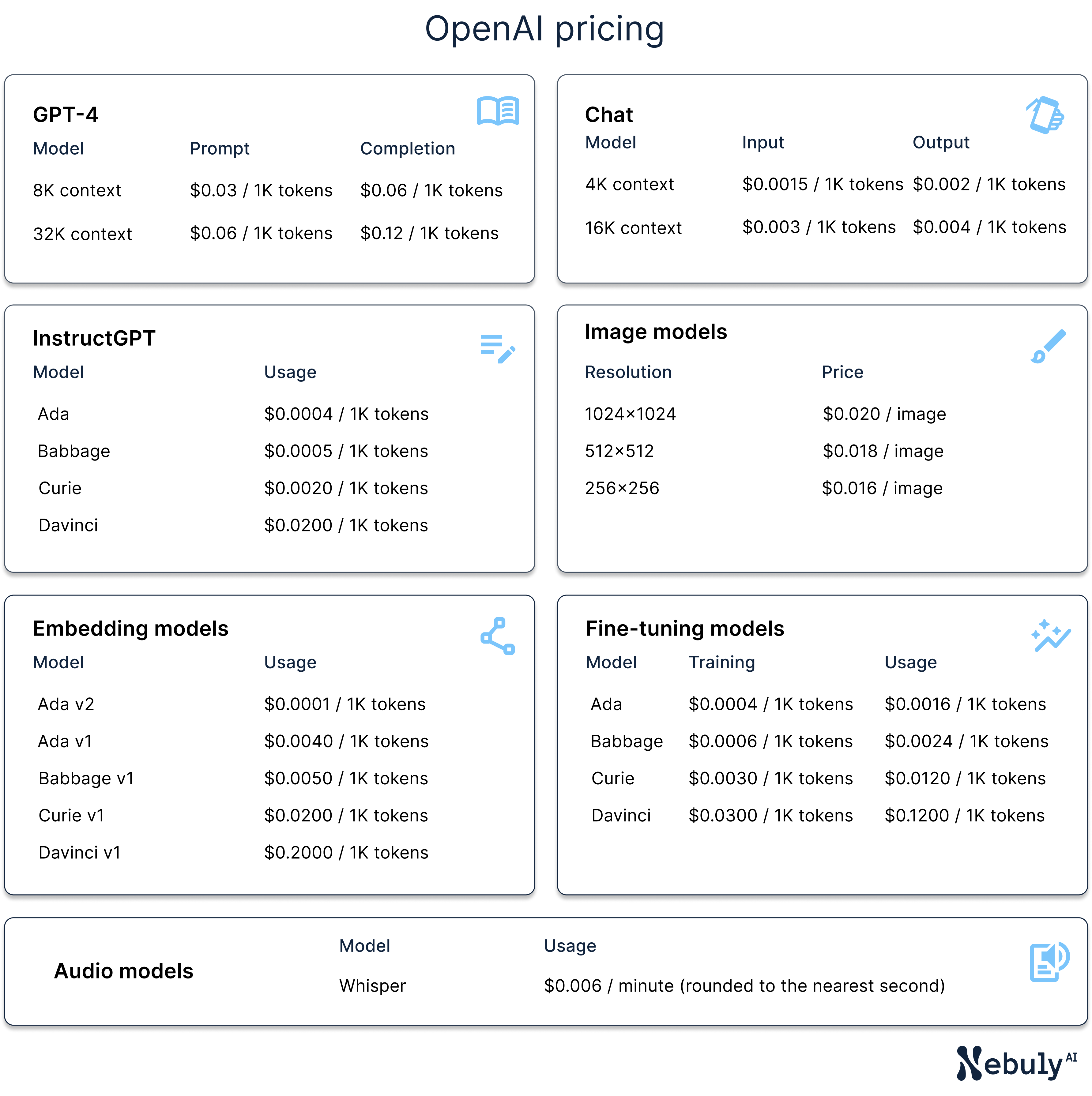
OpenAI also lets users fine-tune, i.e. customize, language models with your own data. While that leads to better and differentiated performances, fine-tuning comes at a significant cost, as the price per token is up to 6x higher than for plain vanilla models.
Upon signing up, users are given a spending limit or quota that can be increased over time as applications demonstrate reliability over time. Users who need additional tokens must ensure that they request a higher quota well in advance of reaching the limit, as the process takes some time. Full details on OpenAI's pricing can be found at OpenAI's pricing webpage.
The complete cost: evaluating the full financial impact of using OpenAI
To paint a more detailed picture of the financial impact of using OpenAI, let's dive into a real use case. We will consider an edtech company - LearnOnline - planning to implement a chatbot to support students Q&A. Since every student can benefit from other students previous questions, a simple chatbot is not sufficient. LearnOnline needs its chatbot to remember previous conversations and therefore designed its AI assistant in the following way:
- The user query is embedded and used to retrieve 5 chats from Pinecone vector database
- The retrieved data, along with the chat history and the user query, are provided as input to OpenAI’s generative model
- OpenAI’s generative model generates the response
As a reminder, embeddings are semantic maps of customer inquiries. Stored in a vector database, embeddings allow the system to remember the context from past chats, overcoming one of the most troublesome limits of conventional chatbots. While there are lots of vector databases (OpenAI cookbook for vector databases), Pinecone’s managed service is a good way to get started as it cuts down time allocation to database setup and upkeep.
In terms of volumes, LearnOnline projects around 40,000 user chats per month. Given an average chat size of 1k tokens (around 750 words or 1.5 pages), this translates into a total consumption of around 40 million tokens per month. Based on empirical evidence, model generated tokens account for 63% of the mix (25 million per month), with user text tokens accounting for the difference (15 million per month). While software costs are expected to represent the largest cost driver, as a US-based company LearnOnline expects to incur significant personnel costs too. Annual salaries are estimated as follows: senior software engineer costs $150k, junior software engineer $100, other IT staff $70k per year.

AI cost evaluation
With this context in mind, let’s drill down into the various cost items:
- Research & consulting phase: the initial step involves establishing project goals, outlining requirements, and devising detailed plans. This task generally falls on the shoulders of an experienced project manager and a lead engineer, who invest 1 month of their time to get the project off the ground. With 2 senior resources involved for 1 month, personnel costs are $25k.
- OpenAI embeddings: OpenAI’s Davinci is a great option when it comes to creating embeddings. The total volume is estimated at 55 million tokens per month, 40m from the full chats plus 15m from input prompts. Given Davinci is priced at $200 per million tokens, or around $1 every 6 pages of text, embeddings costs are $11k per month or $132k per year.
- Pinecone embeddings storage: as a vector database, Pinecone allows to efficiently store embeddings for future use. Pinecone’s p2 pods with 8x size, which are optimized for high performance, are suitable for this application as they can support 8 million saved embeddings, well in excess of estimated volumes. Given an hourly cost of $1.7280, costs are $1.2k per month or $14k per year.
- OpenAI text generation: to ensure cutting-edge mathematical capabilities, LearnOnline has opted for OpenAI’s latest model, GPT4, despite higher variable costs. For the 8K context-window model, input tokens are billed at $0.03 per 1000 tokens, while output tokens cost $0.06 per 1000 tokens. Given GPT4’s asymmetrical pricing in input and output for the 8K context-window model, we need to figure out both token volumes.
Given input volumes of 1,115 million tokens (detail at the end of the article) and GPT4’s pricing of $30 per million tokens, monthly costs are $33.5k. On the output side, volumes are 25 million tokens and unit prices $60 per million tokens resulting in monthly costs of $1.5k. In total, generation API costs are therefore $35k per cost or $420k per year, representing by far the single largest cost item. - Development & integration: a team of 2 junior software developers designs, trains, and integrates the chatbot with the edtech platform. This process usually takes 1 month, resulting in costs of $20k.
- Testing & quality assurance: before going live, the chatbot undergoes rigorous testing to ensure accurate responses and robust error handling. A quality assurance engineer might spend a month on this task, costing around $6k. Including miscellaneous expenses, like software tools for testing and bug tracking, total costs get to $10k.
- Deployment & monitoring: after going live, performance needs to be monitored on an ongoing basis to ensure optimal customer service. An IT specialist could handle this on a part-time basis, leading to an annual cost of about $5k.
- Maintenance & updates: the chatbot needs regular updates and maintenance, including troubleshooting, accommodating new products, and policy updates. A maintenance engineer could manage this part-time, costing about $15k per year.
All-in, LearnOnline needs to invest $640K, most of which is going to be a recurring cost in subsequent years. As shown in the waterfall chart below, OpenAI’s generative API represents the largest single cost item (65%). Nevertheless, other costs - including embeddings and Pinecone - are non insignificant and neglecting them would result in costs shooting 50% above budget. The TCO approach helps overcoming this limitation, allowing businesses to make better informed decisions about their AI investments.
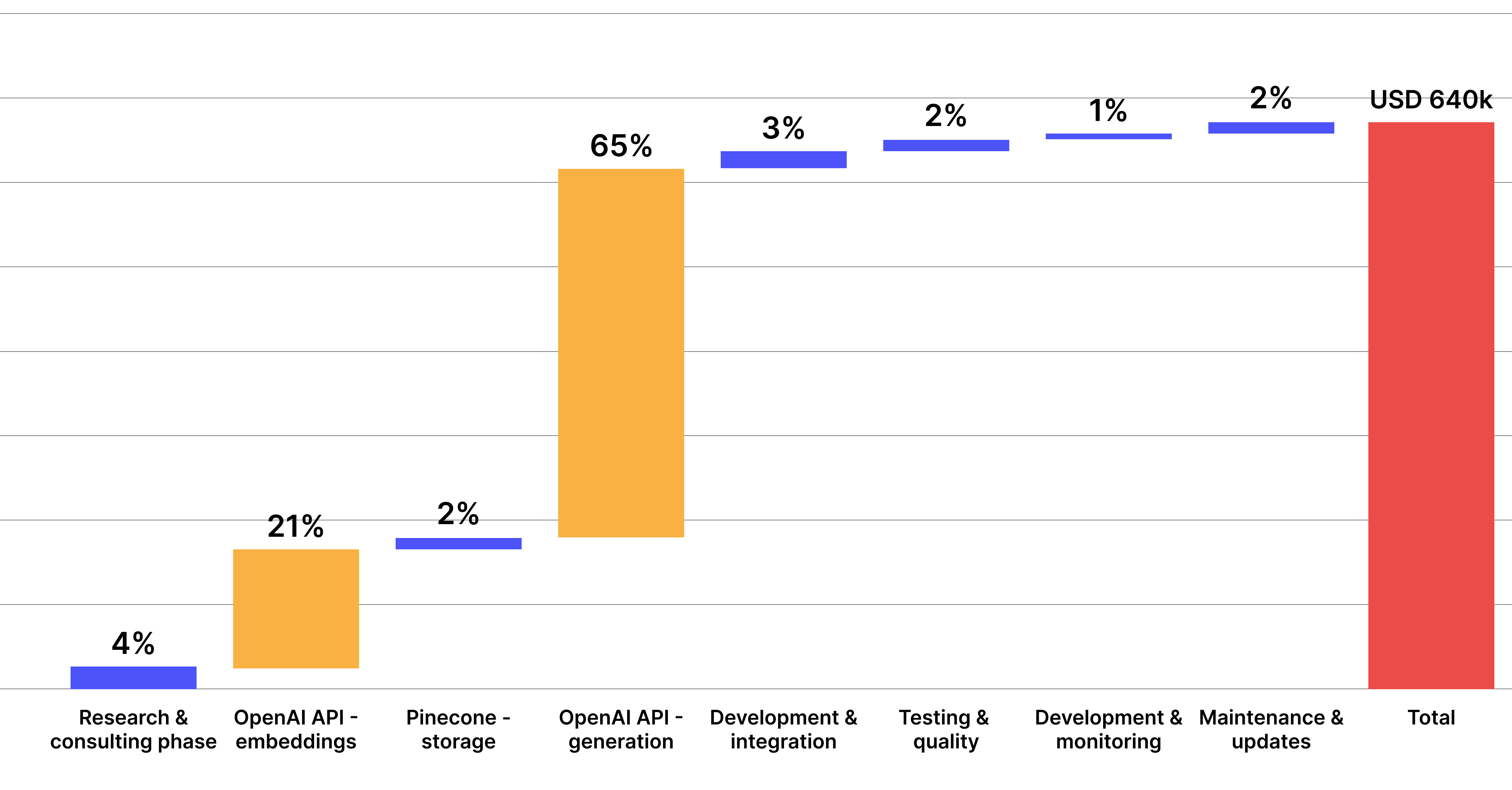